Findings underscore the need to track individuals affected by machine learning predictions
5:00 AM
Author |

Models built on machine learning in health care can be victims of their own success, according to researchers at the Icahn School of Medicine and the University of Michigan.
The study assessed the impact of implementing predictive models on the subsequent performance of those and other models.
Their findings—that using the models to adjust how care is delivered can alter the baseline assumptions that the models were “trained” on, often for worse—were detailed in Annals of Internal Medicine.
“We wanted to explore what happens when a machine learning model is deployed in a hospital and allowed to influence physician decisions for the overall benefit of patients,” said first and corresponding author Akhil Vaid, M.D., clinical instructor of Data-Driven and Digital Medicine, part of the Department of Medicine at Icahn Mount Sinai.
“For example, we sought to understand the broader consequences when a patient is spared from adverse outcomes like kidney damage or mortality. AI models possess the capacity to learn and establish correlations between incoming patient data and corresponding outcomes, but use of these models, by definition, can alter these relationships. Problems arise when these altered relationships are captured back into medical records.”
The study simulated critical care scenarios at two major health care institutions, the Mount Sinai Health System in New York and Beth Israel Deaconess Medical Center in Boston, analyzing 130,000 critical care admissions. The researchers investigated three key scenarios:
1. Model retraining after initial use
Current practice suggests retraining models to address performance degradation over time.
Retraining can improve performance initially by adapting to changing conditions, but the Mount Sinai study shows it can paradoxically lead to further degradation by disrupting the learned relationships between presentation and outcome.
2. Creating a new model after one has already been in use
Following a model’s predictions can save patients from adverse outcomes such as sepsis.
However, death may follow sepsis, and the model effectively works to prevent both. Any new models developed in the future for prediction of death will now also be subject to upset relationships as before.
Since we don't know the exact relationships between all possible outcomes, any data from patients with machine-learning influenced care may be inappropriate to use in training further models.
3. Concurrent use of two predictive models
If two models make simultaneous predictions, using one set of predictions renders the other obsolete. Therefore, predictions should be based on freshly gathered data, which can be costly or impractical.
“Our findings reinforce the complexities and challenges of maintaining predictive model performance in active clinical use,” says co-senior author Karandeep Singh, M.D., associate professor of Learning Health Sciences, Internal Medicine, Urology, and Information at the University of Michigan.
“Model performance can fall dramatically if patient populations change in their makeup. However, agreed-upon corrective measures may fall apart completely if we do not pay attention to what the models are doing—or more properly, what they are learning from.”
“We should not view predictive models as unreliable,” said co-senior author Girish Nadkarni, M.D., M.P.H., Irene and Dr. Arthur M. Fishberg Professor of Medicine at Icahn Mount Sinai, director of The Charles Bronfman Institute of Personalized Medicine and system chief of Data-Driven and Digital Medicine.
“Instead, it's about recognizing that these tools require regular maintenance, understanding, and contextualization. Neglecting their performance and impact monitoring can undermine their effectiveness. We must use predictive models thoughtfully, just like any other medical tool. Learning health systems must pay heed to the fact that indiscriminate use of, and updates to, such models will cause false alarms, unnecessary testing, and increased costs.”
“We recommend that health systems promptly implement a system to track individuals impacted by machine learning predictions, and that the relevant governmental agencies issue guidelines,” said Vaid.
“These findings are equally applicable outside of health care settings and extend to predictive models in general. As such, we live in a model-eat-model world where any naively deployed model can disrupt the function of current and future models, and eventually render itself useless.”
The remaining authors are Ashwin Sawant, M.D.; Mayte Suarez-Farinas, Ph.D.; Juhee Lee, M.D.; Sanjeev Kaul, MD; Patricia Kovatch, BS; Robert Freeman, RN; Joy Jiang, BS; Pushkala Jayaraman, MS; Zahi Fayad, PhD; Edgar Argulian, MD; Stamatios Lerakis, M.D.; Alexander W Charney, M.D., Ph.D.; Fei Wang, Ph.D.; Matthew Levin, M.D., Ph.D.; Benjamin Glicksberg, Ph.D.; Jagat Narula, M.D., Ph.D.; and Ira Hofer, M.D.
Paper cited: “Implications of the Use of Artificial Intelligence Predictive Models in Health Care Settings: A Simulation Study.” Annals of Internal Medicine. DOI: 10.7326/M23-0949
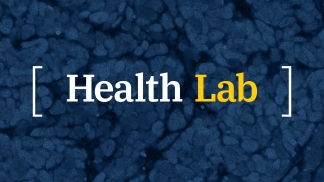
Explore a variety of health care news & stories by visiting the Health Lab home page for more articles.
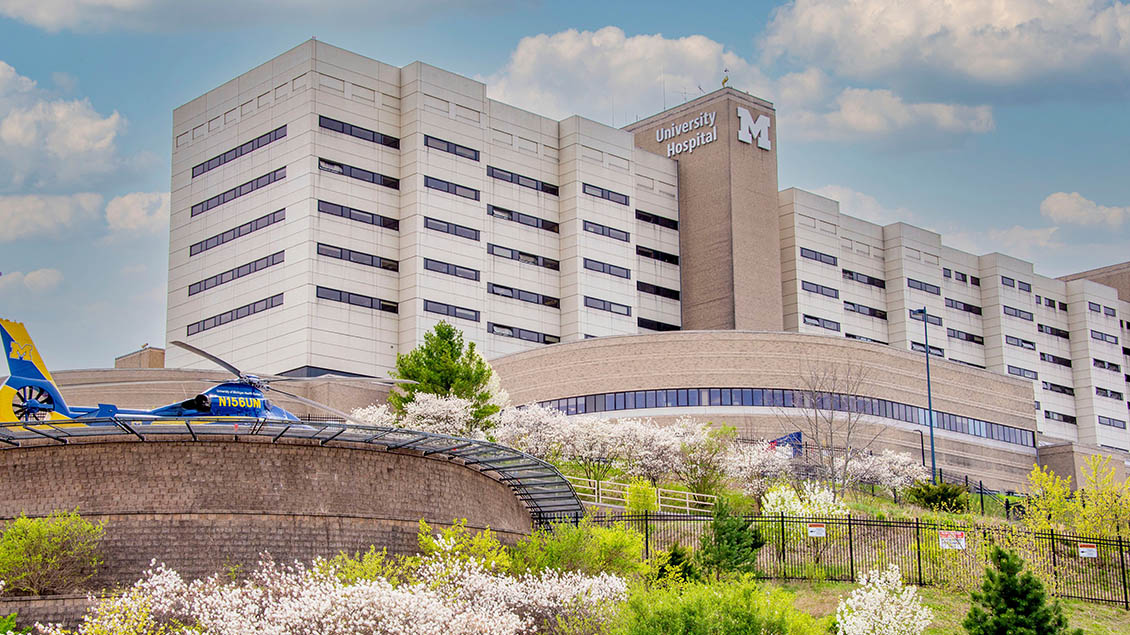
Department of Communication at Michigan Medicine
Want top health & research news weekly? Sign up for Health Lab’s newsletters today!




