An Interview with Dr. Jason Moore
5:00 AM
In this episode, Matt and Donovan talk with Dr. Jason H. Moore, Director of the Center for Artificial Intelligence Research and Education (CAIRE) and Chair of the Department of Computational Biomedicine at Cedars-Sinai Medical Center. Jason discusses the coming impact of artificial intelligence on a spectrum of Alzheimer’s disease and related dementia (ADRD) issues. We discuss how tools such as AI-powered chatbots may improve quality of life for people living with dementia (and their caregivers) and how AI may contribute in the future to diagnosis and treatment.
More resources
Faculty Bio: https://researchers.cedars-sinai.edu/Jason.Moore
Center for Artificial Intelligence Research and Education (CAIRE): https://www.cedars-sinai.edu/research/areas/caire.html
Transcript
Matt Davis:
Okay. ChatGPT, write me a paragraph introduction for a podcast episode that will discuss artificial intelligence and dementia.
Chat GPT:
Hello, and welcome to another episode of Minding Memory. Today we are delving into the profound impact of artificial intelligence on the lives of older adults facing the complexities of dementia. Join us as we explore the ways in which AI is becoming a valuable companion, providing innovative solutions to enhance the wellbeing and daily experiences of individuals...
Matt Davis:
Okay. We'll take it from here. In this episode, we'll be discussing the impact of artificial intelligence on dementia identification, and how AI might be used in the future to improve the lives of people living with dementia. Welcome to Minding Memory, a podcast devoted to exploring research on Alzheimer's disease and other related dementias. Here we'll discuss compelling research and talk with leaders in the field about how their work is improving the detection and treatment of dementia. I'm Matt Davis.
Donovan Maust:
And I'm Donovan Maust.
Matt Davis:
We're both researchers and associate professors at the University of Michigan. I'm a PhD with a background in data science.
Donovan Maust:
And I'm a geriatric psychiatrist, so I think a lot about the diagnosis and management of dementia.
Matt Davis:
I'll work to keep Donovan to the minimum use of medical jargon.
Donovan Maust:
And I'll make sure we talk about research with real world applications to patients and caregivers of individuals with dementia.
Matt Davis:
Thanks for joining us and let's get started. Our guest today is Dr. Jason H. Moore. Dr. Moore is a Professor and Chair of the Department of Computational Biomedicine at Cedars-Sinai Medical Center. He leads an active NIH funded program of research focused on the development of artificial intelligence and machine learning algorithms applied to biomedical data. One application is understanding how demographic, genetic, physiologic and environmental factors interact to influence the risk of common diseases such as Alzheimer's disease. Now usually when we introduce our guests, we avoid stats, but noteworthy here is that Dr. Moore has authored more than 600 peer reviewed publications. Jason, welcome to the podcast.
Jason Moore:
Hi everyone, it's great to be here with you.
Matt Davis:
Since the launch of ChatGPT a couple of years ago, it seems like everybody's talking about AI. So before we jump into some of the specifics of AI and dementia, could you give us just your definition of artificial intelligence?
Jason Moore:
Yeah. There are a lot of definitions of AI. My own personal definition is a computer that can perform complex tasks as well or better than a human. An example would be driving a car. Now, of course, there's a lot that goes into that. There are a lot of technical definitions, but that's really what we're looking for and I think ChatGPT exemplifies that because it can write text as good as some or many humans.
Matt Davis:
So often you'll hear people use the terms machine learning and AI. Are those two terms interchangeable or is there a difference between the two?
Jason Moore:
I see an important difference. AI is really a very broad field, and machine learning is a component of AI. Machine learning is really about learning patterns from data that you can use to make decisions or make predictions, and that's a component of AI. AI involves a whole bunch of other things in addition to machine learning, like what we call knowledge engineering. How do you represent knowledge in the literature or on the web to a computer vision, robotics, those are other potential components of AI that are different from machine learning.
Matt Davis:
This is probably well beyond the scope of what we're going to talk about today, but are there different subtypes of AI?
Jason Moore:
Many different subtypes. For example, there's something called expert systems that generate rules from knowledge and data to make decisions. So yes, there are a lot of different kinds of AI. There are a lot of different kinds of machine learning.
Matt Davis:
Is there a term like general AI or strong AI?
Jason Moore:
Yeah. A general AI would be a system that could perform a lot of different kinds of tasks and do that well.
Donovan Maust:
So assuming most of our listeners are not like AI experts or are not obsessed with ChatGPT, kind of in the normal world where are people most likely to be interfacing with AI, whether or not they realize that they are?
Jason Moore:
Well, AI is everywhere now and most people don't realize that they interact with AI on a daily basis. For example, when you use Google Maps, AI is tracking traffic patterns and recommending to you the best routes to take in your car. When you shop on Amazon, it's making recommendations to you about what you might like to buy, and it's analyzing lots of shopping history data and coming up with what it thinks are the best recommendations. That's AI. When you use social media like Facebook, AI is behind the scenes optimizing what you see on social media, what it thinks you're going to want to see, what you're most likely to be interested in and interact with. When you unlock your smartphone, you're showing your face. That facial recognition is a machine learning AI algorithm that is running, that's looking at the pixels in your face and matching that to what it thinks you look like to make a decision about whether to let you into your phone.
Your 401k, your retirement funds are now traded. The stocks behind those funds are traded using AI. AI is increasingly being used to trade stocks because it can do it in many cases better and faster than humans. So we don't realize it, but we're actually immersed in AI as part of our daily lives.
Donovan Maust:
That's incredible. That's definitely more than I would've guessed.
Matt Davis:
But it sounds like so many of those were specific and helpful tasks. When the public talks about AI, they start getting concerned that it's going to think on its own and evolve and take over all the sci-fi movies and things. But it sounds like that's a bit off. Most of the stuff right now going on is specific task oriented, data retrieval, data analysis type things.
Jason Moore:
Yeah. I think that's true. AI is really at the point right now of making our lives easy in many different ways. Just like most of us can't live without Google Maps now. We use it every time we get in the car we plug in our smartphone and we use Google Maps to plot a course. But I think the fear of AI taking over is at this point in time unfounded. That doesn't mean we shouldn't be talking about it. But I don't think AI has advanced enough to the point of having its own thoughts about how things should be done and what the role of humans are in society. So this idea of what you said earlier about general and artificial intelligence. We're still pretty far off I would say from that scenario where an AI's in charge, it's making decisions, it's controlling our lives in a way that is perhaps detrimental to us.
And if I can expand on that just for a minute, I think the biggest risk of AI in my mind is military applications. Militaries around the world have access to lots of funding. They have the motivation to develop AI. They're actively working on AI. So I think that's probably where we have the biggest risk is militaries using AI to make decisions in either in prevention of warfare or an actual warfare where an AI might make a mistake that could have huge ramifications.
Donovan Maust:
Narrow our focus now to dementia since this is a podcast about dementia. Where do you anticipate the first dementia specific applications of AI coming? Would it be around diagnosis or treatment or caregiving or where's their activity in the space?
Jason Moore:
Yeah, I think about two immediate useful applications where the technology is pretty well-developed. I would point to image analysis. So using AI to diagnose Alzheimer's or come up with a prognosis based on a brain image from something like an MRI scan. Those tools, those AI and machine learning tools are pretty sophisticated and can do a really good job of looking at a brain image and detecting the anomalies, the things that aren't supposed to be there and can do so in many cases better than a human can. And there are lots of published peer reviewed studies now documenting the effectiveness of AI at analyzing all sorts of different types of medical images, and some of those tools are now FDA approved and are being used in clinical practice like for diabetic retinopathy. So I think that's a place where we will see immediate advances is in looking at the brain images from patients maybe with early or even advanced Alzheimer's as a way to figure out exactly what's going on in that image.
The second one I would point to, I think ChatGPT has shown us the power of what we call large language models, which are a component of AI and machine learning. And you could imagine chatbots being developed to interact with Alzheimer patients. And I think that technology is coming along and we're going to see a lot of applications in that space. And there are already chatbots being used in clinical practice. We've adopted one here at Cedars-Sinai Medical Center as a way to interact with patients at the very beginning. When they have a concern, they interact with the chatbot. The chatbot asks them questions and then arrives at some diagnosis or conclusion and helps them schedule an appointment. And then when they go see their doctor, the doctor has a summary of what the AI found from the question and answering and an indication of what an initial diagnosis might be so the doctor can get a headstart when they're actually seeing the patient.
So I think those tools have come along really quickly with the large language model technology, and I think we're going to very soon see a lot of useful applications for dealing with older adults with Alzheimer's or dementia.
Matt Davis:
Is it Siri on steroids in terms of its ability to assist in tasks on a daily basis?
Jason Moore:
Yeah. I think that's a good way to put it. I think we've all been waiting for Siri to get a lot smarter now that we have all these tools. But yeah, I think we're all used to interacting with Siri and can imagine a much more intelligent, interactive Siri. And the technology's there to make that happen, and I'm sure Apple's working on that and will release some things very soon. But that's the general ideas that chatbots will in some cases, I don't want to say take the place of, but augment caregivers and family members for patients that are struggling with dementia issues.
Donovan Maust:
For the example, there you mentioned with the patient symptom chatbot. So is the medical center there then actually looking at, so once those patients see the doctor, if doctors arrive at a diagnosis or want additional evaluation, are you all using that information to basically make the chat smarter?
Jason Moore:
Yeah. The company that provides the chatbot is doing that. It will use our data to make the chatbot smarter and more tailored to our patients. Absolutely.
Matt Davis:
I mean, that must get into data governance stuff too. I mean the patients must give it permission to... It probably needs access to a lot of data, right?
Jason Moore:
Well, when a patient downloads the app, they consent to using the app and of course having whatever they say be incorporated into the AI and used by the AI.
Matt Davis:
So while it might be a bit of a stretch, do you think AI will end up assisting in physical functioning?
Jason Moore:
Yeah. I think the chatbot is certainly one tool, one AI based tool that could be useful maybe in a home setting for older adults. But you could also imagine having a room full of sensors that are keeping track of what the the subject is doing, how they're moving, how they're speaking, how they're talking. And an AI could analyze all that data and alert caregivers that a patient might be declining or something might be wrong, or a patient needs attention or collect data for research purposes so we can better understand the progression of the disease. So I think we're going to see a lot of AI-based tools that are used in conjunction with sensors, with wearable devices to monitor patients and help us understand how we can better help those patients, but also understand how the disease progresses.
Donovan Maust:
In the midst of the COVID-19 pandemic, there's a lot of talk about social isolation concern in particular for older adults, for older adults in nursing homes. Do you think there will be advances in a way that are meaningful for patients with dementia in terms of AI providing opportunities for social interaction?
Jason Moore:
Absolutely. I think we all are anticipating that as this technology matures that we're going to move from these simple chatbots to more robotic like entities that we can communicate with verbally where the robot would have facial expressions that we recognize and understand and have a voice and spoken language and be able to talk to us and understand what we say and have a back and forth. I think the technology's emerging very quickly where we're going to see those kinds of tools and incorporated into more robotic like entities that can, I think, serve that purpose of social interaction where there's a lack of a real human to provide that social interaction. And I think those have the possibility of being quite effective.
Matt Davis:
That seems like, I mean, not only the chatbot for a population that really depends on informal caregiving, but that companionship seems like... And that's part of the reason that we wanted to talk to you so much was, people talk about AI so much, it just felt like when you start thinking about older adults with memory issues, there's just a ton of things that it actually could really be useful for. But before speaking with you too, I never really realized or thought that things like Google Maps were, I never thought of those as AI specifically.
Donovan Maust:
It's like interesting to think about training the AI where the individual that it's interacting with has dementia. So the expected response from the human is not going to be... It just makes you think about the training requirements for the AI when the person on the other end is a human with cognitive impairment. Seems like he would have to have a different type of training, just like with humans.
Jason Moore:
Well, for an AI to be a more general AI, you don't want to just train it to perform a certain number of set tasks. What you would hope is if it's a real AI that it would be adaptive. Because every person struggling with dementia is going to be different, have different language capabilities, have different vocabulary, different aptitude for processing verbal signals. So what you would hope is that the AI can hear and understand what the individual, what the human is saying and then internalize that and change its own verbal responses to match what the individual is going through. So for an AI to be what I would say a real AI, it should be adaptive, it should learn, it should get better to each individual human that it's interacting with.
Matt Davis:
Do you think that these technologies are going to be... As they start to get employed proprietary because I did have the thought that thinking about how you could help with caregiving and assisting and companionship, if it was freely available, it could help with inequities and stuff like that. But if it's proprietary, maybe not everybody would have access to them.
Jason Moore:
Well, there are certainly going to be and are companies developing this technology for this specific purpose. And I'm sure those products are going to be expensive and there will be issues around affordability and health insurance and payment, which creates a class of haves and a class of have-nots that don't have access to that technology. And I think the hope is that academics like us would develop open source and freely available tools that for a very low cost could be put together to deliver something that might be almost as good as what a commercial company can provide. But I do think this is something that we as a society are going to have to think a lot about is who has access to this technology and who doesn't.
Matt Davis:
All right. So shifting gears a little bit. We do know that your team has some ongoing projects around AI and Alzheimer's disease. So big picture, can you tell us a little bit what you're working on?
Jason Moore:
Sure. So let me start with the high level motivation. There's a lot of interest in new drugs for Alzheimer's disease because the current selection is not particularly effective. So a lot of my research is focused on how can we identify new genes that are predictive of risk of Alzheimer's disease? And when we find new genes that are predictive, then hopefully those new genes will point to drug targets that we can use as the basis for the development of new drugs. So that's the motivation behind my work is ultimately to provide those new drug targets. So the approach that we're taking is using machine learning to look at genetic variation that's been measured in patients with and without Alzheimer's disease so that we can develop predictive models with the hope of identifying genes.
And I'm a methodologist, so I develop cutting edge new AI and machine learning tools. And we've developed over the last 10 years, some of the very first and most widely used what are called automated machine learning tools. And one of the challenges of machine learning is there are lots of different algorithms, there's lots of different ways to do it. Every algorithm has a lot of different settings, what we call parameters. So it's a very complex task to develop a machine learning model that is predictive of something like Alzheimer's disease just because there's so many decisions that need to be made and you have to have extensive expertise to know how to do that. So the idea of automated machine learning is to get the computer to do that through a higher level AI that can automatically pick the best machine learning algorithms to piece together in a pipeline to do an analysis. So that's what I've been working on the last 10 years is developing AI strategies for automating machine learning, taking the guesswork out.
And the advantage of that is not only does it make your machine learning more powerful, but it makes machine learning technology more accessible to everybody, clinicians, epidemiologists, biologists. You don't have to be a computer scientist necessarily to use these tools because the computer is doing the hard work for you. So that's a major motivation for my work is how do you democratize this powerful technology so as many people as possible have access to it? So that's been a big focus of my work the last 10 years, and we're actively developing those kinds of algorithms to look at data, research data for Alzheimer's disease. But another very important piece of this is that a lot of the machine learning and AI that's being done today with data for data analysis ignores everything we know about the problem. The typical approach is to take a fancy machine learning algorithm, throw it at a lot of data that's been collected and hope that the machine learning algorithm can figure out the patterns that are relevant for your particular question.
But the algorithm doesn't understand anything about Alzheimer's disease or dementia or genes or biology. It's ignorant of all of that knowledge. So a big focus of my work right now is how do we get one of these automated machine learning algorithms to understand the knowledge that's in the literature and to use that to preferentially pick genes and machine learning models and to do interpretation of those models to make the machine learning more relevant for the specific clinical and biological questions that we're asking. So what we've done is assembled a very comprehensive knowledge base about Alzheimer's disease and genes and drugs and biochemical pathways and symptoms, and we've made that publicly available. It's at alzkb.ai. We're developing some more user-friendly interfaces for it, but the knowledge is all there. It's accessible to researchers who want to use it, and we're currently linking all of that knowledge with our automated machine learning tool. So that's a big focus on my work right now.
Donovan Maust:
If you think more generally in the field and advances of AI specifically towards dementia, you already mentioned a couple earlier around say like neuroimaging, but any other big picture developments where you think listeners should keep an eye say over the next three to five years?
Jason Moore:
Well, I think we've covered a lot of the exciting things that are happening right now, especially in the area of large language models. What I would look for is, and what we're actively doing is how do you put all these pieces together? How do you put the fancy machine learning algorithms that are looking at brain images and lots of Alzheimer data together with the large language model technology, together with all the knowledge that we have, together with all the clinical considerations around how you make decisions in the clinic about patients using all of these tool? How do we start to put all those pieces together to create an AI based platform that is easy to use, is intuitive, is effective, fits within a clinical workflow, is trustworthy and cost-effective? So that's I think the challenge. We have a lot of really wonderful tools now. It's really all about how do we piece them together in an effective way to really transform what we're trying to do with people with Alzheimer's disease and dementia.
Donovan Maust:
In a lot of other industries I think there's been a lot of concern related to AI specifically unemployment. And basically that it's like replacing humans. Will it make a geriatric psychiatrist obsolete or will it replace neurologists?
Jason Moore:
Yeah. That's a great question and a question that I think a lot of people are concerned about. I'm in Los Angeles and we just went through the writer and actor strikes this past year, and one of their chief concerns was that they would be replaced by AI. I mean AI can now create video and voice and conceivably replace actors in some situations or writers in some situations, and they were able to negotiate protections for their jobs. So I think it's a valid concern. And in the medical space, clinical space, I think it's a valid concern. But a lot of people like to use the term augmented intelligence rather than artificial intelligence. And the basic idea of augmented intelligence is that AI is really just another clinician in the room with you and there to provide a different opinion or a different suggestion or an alternative view into what's going on with a patient and we as the human caregivers, healthcare providers can either take that what the AI's recommending or not. And in that sense, it's augmenting what clinicians are doing rather than replacing clinicians.
So I think that's the approach that seems to be very popular among both AI experts and clinicians, is this idea of augmenting what we already do. And like I said earlier, I think it's going to be a long time still before AI starts replacing all of the things a clinician can do. The depth of knowledge, the depth of experience, the human intuition that we have, our decision-making capabilities, a lot of the softer sides of what a clinician does, understanding human emotion and how humans think and react to information. I could go on and on, but I think replacing all of that with a computer is going to take a long time. But in the meantime, I think we need to put this focus on augmenting what humans are doing with AI.
Matt Davis:
It's Hollywood's fault because there's always an autodoc in all the sci-fi movies that makes all the decisions. I don't know if it's analogous to this, but I'm really excited about self-driving cars because I'm looking towards when I'm an older adult, I want my car to just drive me to places. And there's resistance to it. I mean, the technology may even be close it seems like. But I wonder if that's how things are going to roll out with medicine or even investing too, even though an AI generated tool for investment or for diagnosis, people are still not going to just take that without the input of a human it seems like.
Jason Moore:
Well, I think what it boils down to is trust. Do you trust getting in a car that's going to drive you somewhere across the city based on AI? And personally, I don't trust self-driving cars right now and would not get in one other than a very short trip for the novelty factor. But as I think about self-driving cars, I think the best when self-driving cars are going to be useful is when all the cars are self-driving, and you take the human error out of the equation. Every time we get behind the wheel and drive somewhere, we're constantly worried about accidents. We're being defensive and worried about what other drivers are doing. So human-based driving is somewhat dangerous and it's somewhat risky. So I think self-driving cars, if all the cars on the road were self-driving and following the same rules and reacting to each other in a very predefined way, it could take a lot of uncertainty and error and randomness out of the driving process that we as humans have to deal with.
Matt Davis:
So I have a son in college right now who's thinking about careers. So I'm just curious, based on your background, thinking about the future in AI in a very general and broad sense, what would you recommend people think about in terms of the careers of the future and living side by side with AI?
Jason Moore:
Well, I think first and foremost, I think we as humans have to learn about AI, understand AI and what it's doing if we're going to live in a world with AI. And the more each of us understands AI, the better we're going to be able to both interact with it, but also understand its strengths and weaknesses to live side by side with AI. So I think AI is going to permeate virtually every career that somebody could choose, just as we've seen with the actors and the writers that we talked about, AI is permeating that industry, and I think it's important that no matter what your career path, that you have some base understanding of AI and what it can do and what it can't do, and think about how to use AI to your advantage.
I mean, that's really what the next 10 years is going to be about in every career path is, okay, how can I harness this technology to make my particular job easier, faster, more effective, enrich what I'm doing, again, augment it, not replace it, but how can I use it to enrich what I'm doing? But that requires some knowledge of AI to be able to do that and to be able to do that effectively. I mean, we've seen that with ChatGPT. There are endless how-tos now on how to engineer prompts to get ChatGPT to do what you need it to do, whether you're writing poetry or writing for a scientific journal or summarizing text or using it to write computer code, which it's very good at now. So you have to learn how to interact with the AI to use it effectively. So that's what I would say is, learn about AI and think about how you can use it in your own career path, whatever that might be.
Matt Davis:
That response touched on something that we actually didn't really discuss so far, and that's ethics, I think, in terms of the ethical use. We're thinking about in academics like people using AI to generate work that may not represent what the student did. I have to say, I was at a meeting not too long ago where all about AI and talking about it and what does it mean for those of us teaching at the university? And then afterwards, it dawned on me, there was no one ever mentioned anything about ethics. And I feel like almost like that needs to precede ethical use of AI. And I don't know, thinking about also us as a country developing AI to keep in pace with other countries and stuff. I don't know, it feels like that should be part of this conversation, using it for the right things that sometimes doesn't come up because people get so excited about the technology. I don't know if you have any thoughts about that.
Jason Moore:
Yeah. I'm glad you mentioned that, Matt. I think about this every day. It's something that working in a hospital we think about all the time. I mean, imagine putting a robot in a home environment with an elderly person to provide that social companionship and maybe to collect data about that patient. Imagine if that robot harmed that individual in some way? Who's at fault? Is the robot at fault? Is the elderly person at fault? Is the company that developed the robot and sold it to the individual at fault? Is the clinician at fault that allowed this to happen with their patient? Is the health insurance company at fault that paid for it? Who's at fault? So that's an important ethical issue that's going to have to be sorted out. I'm in the State of California and there's currently legislation that's being considered to regulate AI in clinical settings.
And one of the pieces of legislation says that if we use AI as part of a patient's care, that we will have to reveal that to the patient. So imagine telling a patient, AI is going to be used for X, Y, and Z as part of your clinical care. How are patients going to respond to that? Are they going to feel comfortable with that? Are they going to trust it? Can they say, no, I don't want AI involved? And then how does a hospital respond to that? How do they turn off AI that's now embedded in everything they do? So there are a lot of legal issues around AI. I heard a fascinating lecture recently from a University of California lawyer who is thinking about AI in the courtroom and how right now judges are not allowing attorneys and defense teams to look under the hood of AIs that have been used to evidence on a client.
So you can't look at the source code and see what the AI is doing. Do you trust it? Is it making mistakes as part of a defense strategy? That seems like a big issue that needs to be addressed. So as this area moves very quickly, there are tons and tons of ethical and legal and social issues around AI in the clinic, outside the clinic that we are going to have to tackle head on as a society.
Matt Davis:
So my last question, I guess for the podcast team is do we think we should keep using ChatGPT for our podcast intros?
Donovan Maust:
No. Yours are better, man.
Matt Davis:
Well, thank you so much. When I was playing with it, I must admit, this was my first time playing with ChatGPT. It was wordy. I kept having to tell it to go shorter and shorter, write only a three sentence introduction. But it did a pretty nice job I thought, in terms it sounded a little bit glorious our podcast episode.
Jason Moore:
What's interesting is that humans are pretty good at detecting when ChatGPT has been used to write something or say something. It's just a little off whatever it's doing. It's just a little bit different from how we as humans talk and write. So in a lot of cases, it's not that hard to figure out that ChatGPT has been used.
Matt Davis:
Well, this has been really fascinating. Jason, thanks so much for joining us, and thanks to all of you who listened in this season. If you enjoyed our discussion today, please consider subscribing to our podcast. Other episodes can be found on Apple Podcasts, Spotify, and SoundCloud, as well as directly from us at capra.med.umich.edu, where a full transcript of this episode is also available. On our website, you'll also find links to our seminar series and the data products we've created for dementia research. Music and engineering for this podcast was provided by Dan Langa. More information available at www.danlanga.com. Minding Memory is part of the Michigan Medicine Podcast Network. Find more shows at uofmhealth.org/podcasts. Support for this podcast comes from the National Institute on Aging at the National Institutes of Health, as well as the Institute for Healthcare Policy and Innovation at the University of Michigan. The views expressed in this podcast do not necessarily represent the views of the NIH or the University of Michigan. Thanks for joining us, and we'll be back soon.

Listen to more Minding Memory podcasts - a part of the Michigan Medicine Podcast Network.
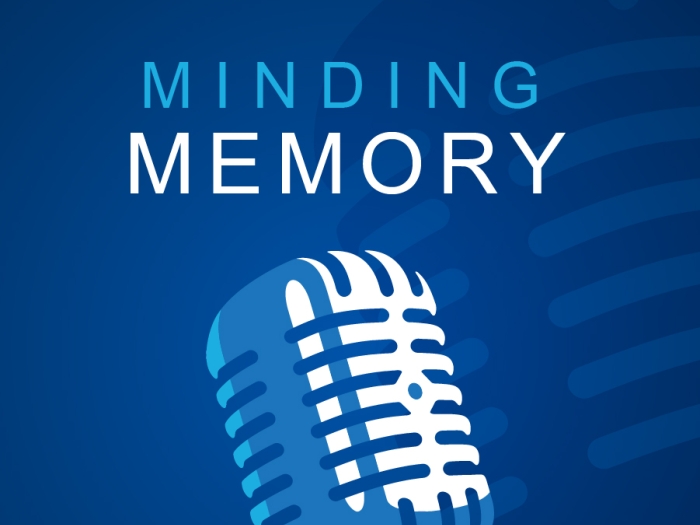
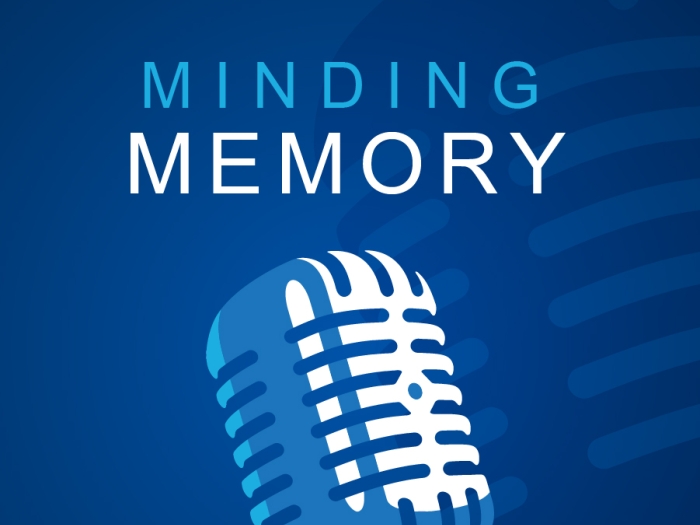


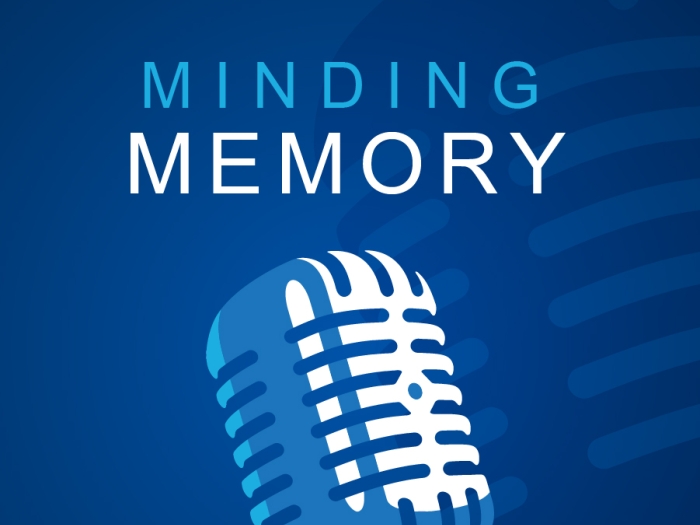
